Featured Paper
Self-supervised representation learning for nerve fiber distribution patterns in 3D-PLI
By Alexander Oberstrass
date: Nov 26 2024
Quantifiable and interpretable descriptors of nerve fiber architecture at microscopic resolution provide an important foundation for a deeper understanding of human brain architecture. 3D polarized light imaging (3D-PLI) is a microscopic imaging technique capable of resolving the course and geometry of nerve fibers in whole postmortem brain sections represented in large data sets. However, analysis of 3D-PLI images is challenging due to the large volume of data and complex textures, which means a significant effort in creating data annotations.
To this end, we recently conducted a study on the capabilities of self-supervised learning for automatic characterization of fiber architecture in 3D-PLI, now published as an article in Imaging Neuroscience. In this article, we employed a specific self-supervised learning strategy that leverages volume reconstructions of individual and independently measured brain sections to sample positive pairs for contrastive learning. Pairs are identified based on a fixed distance between small sample image patches across brain sections in a 3D context (CL-3D). The efficacy of this approach is demonstrated on the occipital lobe of a vervet monkey brain. Axes of maximum variance in CL-3D feature space are shown to align well with microstructural organizational principles, such as gray and white matter, myeloarchitectonic layer structures, fiber bundles, crossings, and fannings. Features fall into spatially consistent clusters of similar fiber architecture (see Figure 10), demonstrating robustness to variations between independently acquired brain sections when learning in a 3D context. Furthermore, CL-3D embeddings require less manual annotation to achieve convincing tissue segmentation compared to other texture descriptors.
Without any supervisory signal, CL-3D features form a well-structured embedding space that can be beneficial for various downstream tasks in 3D-PLI analysis, including data exploration, query-based retrieval of selected template structures such as U-fibers and mapping of fiber architecture with minimal labels. While demonstrated for a 3D-PLI dataset, the proposed CL-3D method can potentially be applied to other microscopic modalities in other brains with available 3D reconstructions, as well.
The full article is now available in Imaging Neuroscience: https://doi.org/10.1162/imag_a_00351
Extracted features and clusters are available for download on EBRAINS: https://doi.org/10.25493/78A4-KTU
Code for training the CL-3D model is available on GitHub: https://github.com/FZJ-INM1-BDA/cl-3d
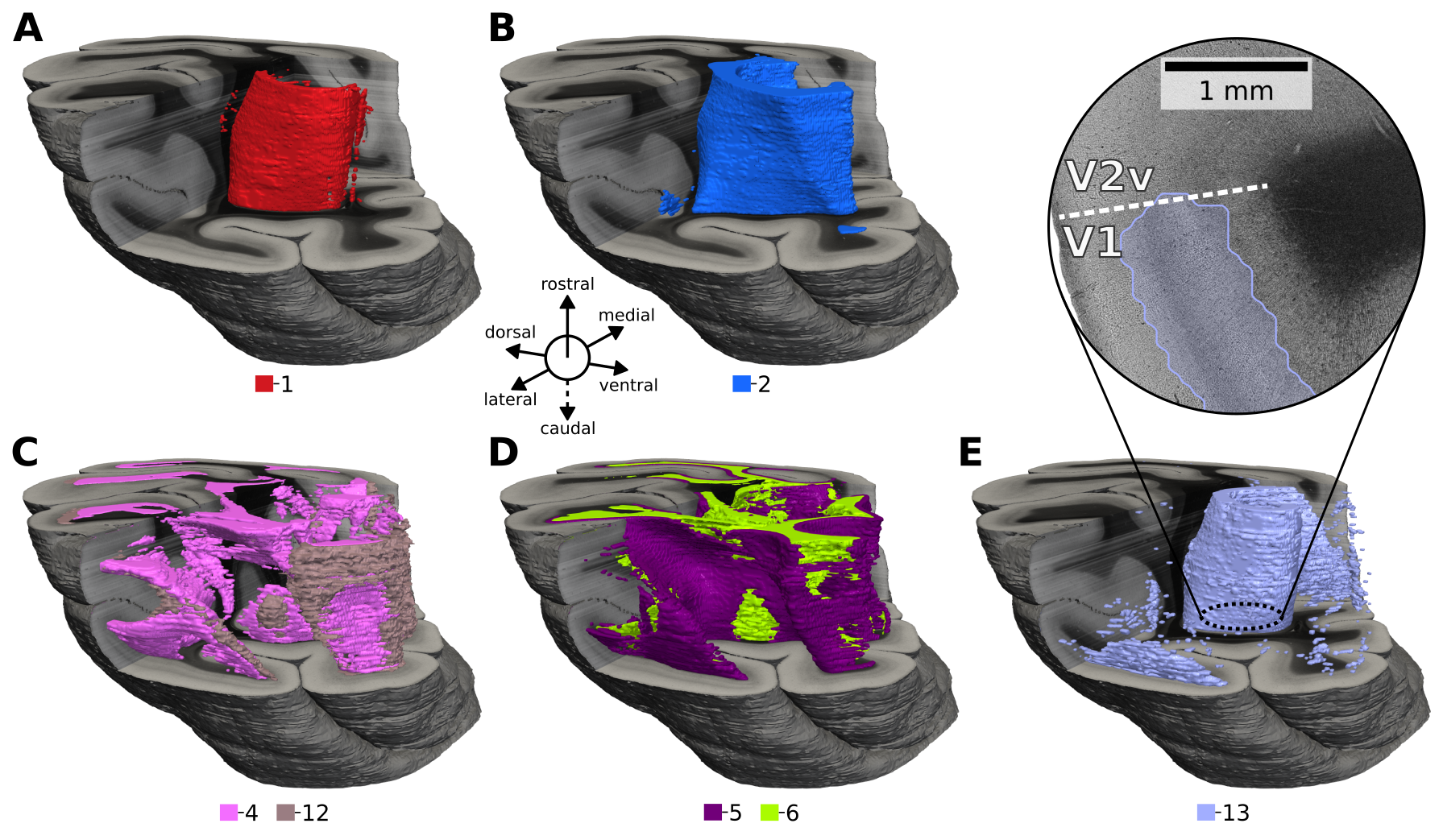
Figure 10 from the paper. Clustering results of CL-3D features on the 3D reconstructed occipital lobe. Clusters highlight approximately (A) the tapetum and stratum calcarinum (1), (B) steep fibers of the sagittal stratum (2), (C) close to in-plane twisting or crossing fibers (4) and flat fanning fibers (12), (D) flat fibers for layer VI (5) and white matter (6), (E) mainly layers IVb-V of primary visual area V1 (13).